Dropbox’s shares have fallen -27.6% from its July high of $26.20, which was under the recent February high of $26.49 as of 09/06/2019. It’s also -57.75% under the ATH of $43.50, so I saw this as a perfect opportunity to leverage R and run a ‘Quick N’ Clean’ valuation to answer a couple questions like ‘What sets Dropbox Apart’ and more.
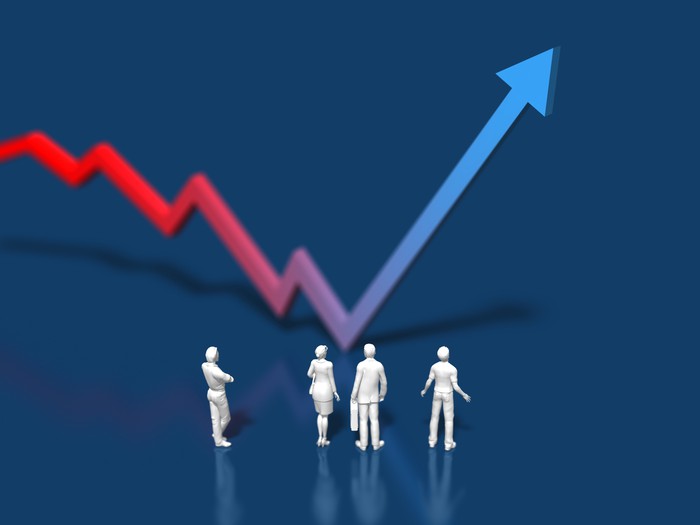